CERN Living Lab
CERN Living Lab
Project goal
The project goal is to develop a big-data analytics platform and tools for large-scale studies of data under special constraints, such as information that is privacy-sensitive, or that has a varying level of quality, associated provenance information, or signal-to-noise ratio. Ethical considerations are also considered when necessary. This will serve as a proof-of-concept for federating and analysing heterogeneous data from diverse sources, in particular for medical and biological research, using ideas and expertise coming from CERN and the broader high-energy physics community.
Collaborators
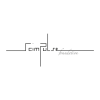
 (1).png)
Project background
CERN is a living laboratory, with several thousand people coming to work at its main campuses every day. For operational purposes, CERN collects data related to health, safety, the environment, and other aspects of daily life at the lab. Creating a platform to collate and enable intelligent management and use of this data — while respecting privacy and other ethical and legal obligations — offers the potential to improve life at the lab. At the same time, such a platform provides an ideal testbed for exploring new data analytics technologies, algorithms and tools, including machine-learning (ML)/deep-learning (DL) methods, encryption schemes, or block-chain-based ledgers. It also provides a natural bridge to collaborate with other scientific research domains, such as medical research and biology.
This project is being carried out in the context of CERN's strategy for knowledge transfer to medical applications, led by CERN's Knowledge Transfer group.
Recent progress
In 2020, the project activities focused mainly on the investigation of privacy-preserving techniques for data analysis, particularly in cases where machine-learning or deep-learning models are used. A systematisation of the state-of-the-art was conducted looking at different methodologies, such as homomorphic encryption, secure multi-party computation and federated learning. The existing implementations and their capabilities were assessed against reference use cases, including the extraction of features from brain MRI scans and aggregated data classification for epidemiological research. In 2020, two new collaborators joined the initiative: the University of Madrid, Spain, and the Seoul National University Bundang Hospital (SNUBH), South Korea, sharing expertise in security and the analysis of medical data.
Next steps
Presentations
- T. Aliyev, Meaningful Control of AI and Machine Ethics (7 June). Presented at Big Data in Medicine: Challenges and Opportunities, CERN, Geneva, 2019. cern.ch/go/J7CF
- A. Di Meglio, The CERN Living Lab Initiative (20 June). Presented at CERN Information Technology for the Hospitals, HUG, Geneva, 2019. cern.ch/go/Fld8
- T. Aliyev, Interpretability and Accountability as Necessary Pieces for Machine Ethics (2 July). Presented at Implementing Machine Ethics Workshop, UCD, Dublin, 2019. cern.ch/go/7c6d
- A. Di Meglio, The Living Lab Project (23 January). Presented at CERN openlab Technical Workshop, CERN, Geneva, 2020. cern.ch/go/Cf7R