Future technologies for medical Linacs (SmartLINAC)
Future technologies for medical Linacs (SmartLINAC)
Project goal
The ‘SmartLINAC’ project aims to create a platform for medical and scientific linear accelerators that will enable anomaly detection and maintenance planning. The goal is to drastically reduce related costs and unexpected breakdowns. The platform we develop will use artificial intelligence to adapt itself to different linear accelerators (Linacs) operated in all kinds of environments.
Collaborators
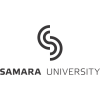
Project background
During a joint workshop held at CERN in 2017, involving the International Cancer Expert Corps and the UK Science and Technology Facilities Council, the need for simple-to-maintain-and-operate medical Linacs was emphasised strongly. Maintenance can be one of the main sources of expenditure related to Linacs; it is essential to reduce this cost in order to support the proliferation of such devices.
Following contacts with Samara National Research University in Russia in 2018, it was decided to create the SmartLINAC project. The university has a long history in the field of aerospace, which requires similar attention to fine detail and has led to the building up of expertise in big-data processing.
This project is being carried out in the context of CERN's strategy for knowledge transfer to medical applications, led by CERN's Knowledge Transfer group.
Recent progress
Following work to define the project’s scope in 2018, as well as an initial feasibility study, the main project got underway in 2019. For the first stages of development within the project, data has been used from the Linac4 accelerator at CERN. In particular, we have used data from the 2 MHz radio-frequency source that is used to create the plasma; this presents periods of ‘jitters’ that influence the beam’s quality.
By nature, these data sets are extremely noisy and volatile, leading to difficulties in interpretation and labelling. Therefore, the first research objective was to establish an appropriate data-labelling technique that would make it possible to identify ‘jittering’ periods. This has led to the creation of an anomaly detection system that recognises early symptoms in order to make preventive maintenance possible. Several approaches based on statistics and neural-network technologies were used to solve the problem. These approaches are now being combined in order to offer a system that can be adapted to different sources.
The data has been shown to be extremely difficult for neural networks to categorise. Rather than using neural networks to detect anomalies themselves, we have therefore made use of them to define appropriate parameters for a statistical treatment of the data source. This will, in turn, lead to detection of anomalies.
Next steps
Publications
- Y. Donon, Smart Anomaly Detection and Maintenance Planning Platform for Linear Accelerators (3 October). Presented at the 27th International Symposium Nuclear Electronics and Computing (NEC’2019), Montenegro, 2019. cern.ch/go/nb9z
Presentations
- Y. Donon, Smart Anomaly Detection and Maintenance Planning Platform for Linear Accelerators (3 October). Presented at the 27th International Symposium Nuclear Electronics and Computing (NEC’2019), Montenegro, 2019.
- Y. Donon, Anomaly detection in noised time series: the challenge of CERN’s LINAC4 (24 January). Presented at The Open Data science meetup #3, Samara, 2020. cern.ch/go/9PZD